Angela Zhou
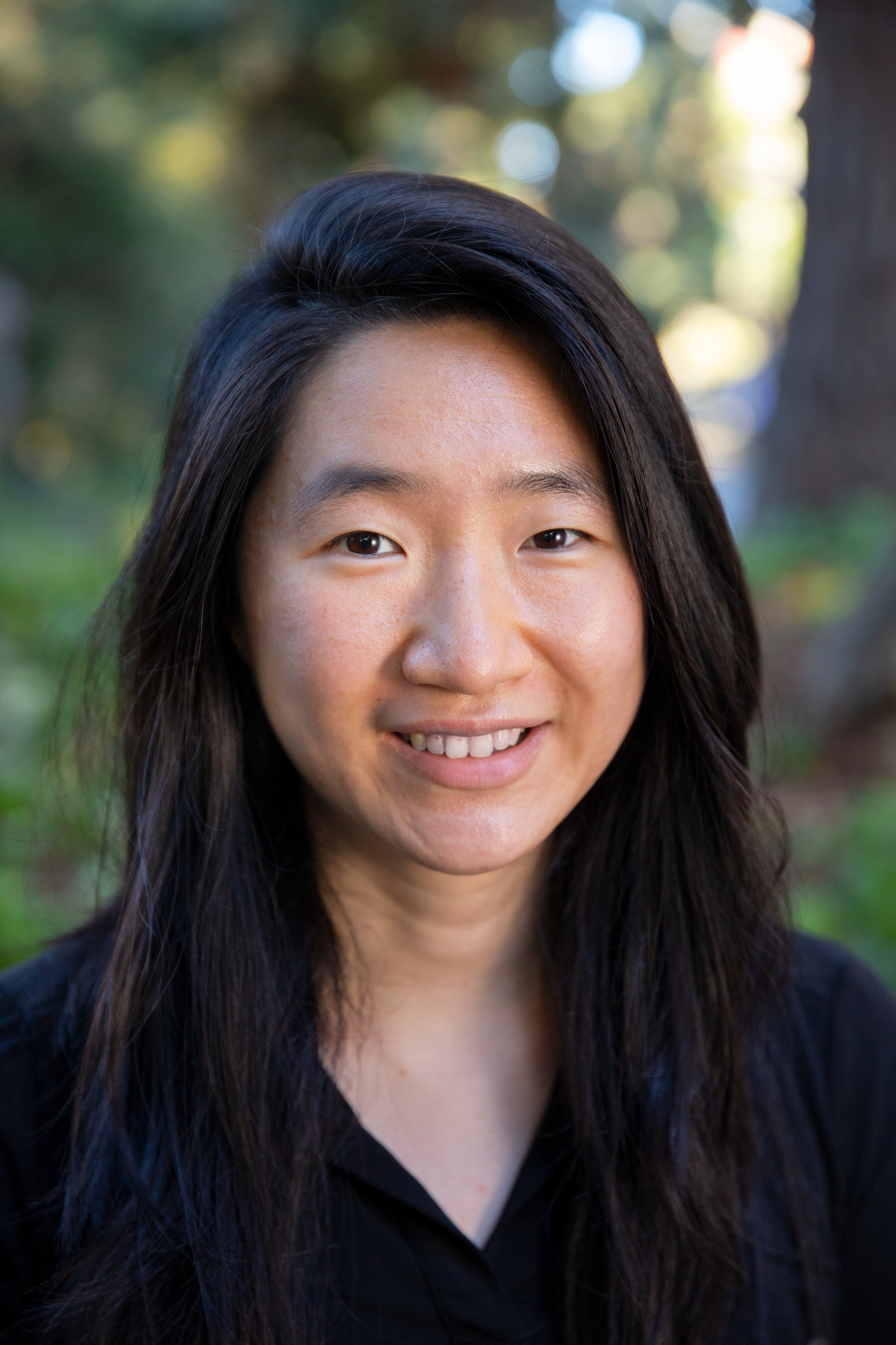
401S Bridge Hall
I am an Assistant Professor at USC Marshall Data Sciences and Operations, in the Operations group.
Previously I was a research fellow at the Simons program on causality and a FODSI postdoc at UC Berkeley. I obtained my PhD from Cornell University in Operations Research and Information Engineering working with Nathan Kallus at Cornell Tech. My work was previously supported on a NDSEG fellowship.
My research interests are broadly in data-driven decision making under uncertainty, including operations, statistical machine learning, and causal inference, and the interplay of statistics and optimization.
Email: zhoua at usc.edu.
Interested in working with me? Advising/mentorship plan and FAQ
Research interests by topic:
- Computerized influence functions for optimization-based estimators in causal inference
- Program evaluation perspective on algorithmic accountability; equity and efficacy in the provision of social services: fair/optimal encouragement designs
- Credible causal inference for optimal decisions and bounds; and for infinite-horizon and finite horizon (scalable) offline reinforcement learning under violations of assumptions
- Algorithmic fairness: biased data, partial identification bounds on causal disparities. Substantive: don’t use CJ data for ML benchmarks, evaluating short term impacts of bail reform on aggregate crime.
selected publications
news
Jan 19, 2024 | My paper on Reward-Relevant-Filtered Linear Offline Reinforcement learning was accepted at AISTATS 2024! (journal version under preparation) |
---|---|
Sep 21, 2023 | Delighted to share that my paper on Optimal and Fair Encouragement Policy Evaluation and Learning is accepted at Neurips 2023! TL;DR: under incomplete take-up, we care both about utility and who gets access to services. Let’s work together to equitably reduce administrative burdens. See you in New Orleans! |
Sep 21, 2023 | Big update to Robust Fitted-Q-Evaluation and Iteration under Sequentially Exogenous Unobserved Confounders, with new and exciting results on complex healthcare data and warm-starting RL! |
Sep 20, 2023 | Thanks Microsoft for the Accelerating Foundation Models award! |
Aug 30, 2023 | Check out our report on EAAMO 2022 in SIGecom Exchanges! |
Aug 23, 2023 | I’ll be an Area Chair for AISTATS 2023. |
Aug 10, 2023 | New paper posted on Optimizing and Learning Assortment Decisions in the Presence of Platform Disengagement! (with Mika Sumida) |
Jul 1, 2023 | Our paper on An Empirical Evaluation of the Impact of New York’s Bail Reform on Crime Using Synthetic Controls was accepted at the journal of Statistics and Public Policy! |
Jun 1, 2023 | Major (working) update on Data-Driven Influence Functions for Causal Inference and Optimization-Based Estimators, with new results on sensitivity analysis! |
Apr 13, 2023 | Upcoming talks at USC ShowCAIS (April), USC Econometrics Reading group (April), Simons (Causality reunion) (May), Socal OR/OM Day (May), Stanford OIT (June), MSOM |